Predictability in sales and marketing, and the problems of it
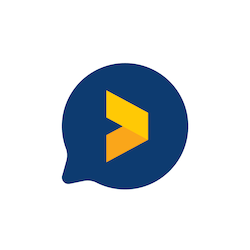
Last edited: March 27th, 2024
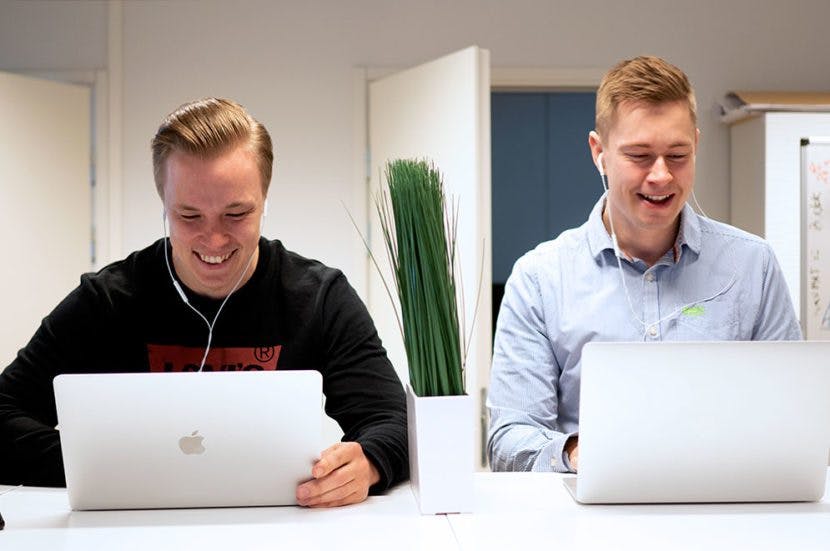
Predictive analytics and all sorts of predictions are quite trendy in marketing and in business in general.
People are trying to predict and understand the future of a company, by using all sorts of patterns and fancy sounding techniques.
The idea of this blog text is not to give you the final answer to the question on how to predict the future of your business. The goal is to offer the tools for understanding, what are the things you should even be trying to predict, and what types of problems are related with prediction.
With e-commerce stores it is a big trend to talk about the customer lifetime value, and how to optimize it. In some cases, they have gone as far as talking about allocating the marketing budget based on what campaigns are producing the best customers measured by the customer lifetime value.
In a sales organization common subject for discussion is the predictability of sales. Often this predictability of sales is focused on very basic factors:
- Make an X-amount of phone calls to obtain Y-amount of meetings.
- Agree on the Y-amount of meetings, and close Z-amount of sales
The amount of sales varies a lot on a monthly basis, especially in the small businesses.
The amount of phone calls and agreed meetings will give a picture of what to expect, if you are heading towards the right direction and how much deals will be closed during the next few days, weeks and months.
Of course the correlations between different subjects are stronger or weaker, depending on the business, but the basics are always the same. The same problems will emerge, no matter what type of business we are talking about.
The challenges and impossibilities of predicting
The inspiration for this whole blog came originally from a tweet from Jaffer Ali:
”It does not take an abundance of brain power to understand, that the more volatile and unstable the road ahead is, the less one can run one’s car looking in the rear view mirror.”
This tweet is in my opinion one of the best summaries of the idea on what can be predicted, and at what situations it is better to stop looking at that back mirror and making assumptions based solely on that.
Not to jump in too deep, let’s start with the basics of predicting, and the main problem with it, the problem of induction.
I haven’t read enough of philosophy to know the origin of this problem too well, but at least many times when talking about this problematic it is common to refer to David Hume and his thoughts on the matter.
If you want to summarize the problematic, you can describe it with the following example:
- 100 million sightings of white swans does not guarantee the fact that all swans are white.
- However, one sighting of a black swan guarantees the fact that not all swans are white.
You can read a lot more about this problematic online, and try to figure out the deepest meaning it holds for the predictability on your late night walks. But at this point, try to take with you at least this one main point about the issue:
- Predictions made based on historical data will never achieve a 100% certainty. And the size of your sample, won’t change this fact.
No matter what type of predictions you make, and no matter how statistically relevant they are, you should always be prepared that there is going to appear something, that you didn’t count for.
Through this it is easy to carry on looking more deeply into the challenges of predicting, because when put to practice all predicting is more or less based on using historical data. But we are never capable of making predictions that would come to reality with a 100% certainty.
How likely predictions are we capable of making?
Often when starting from the problem of induction, the next question related to predicting is ”Aren’t we getting almost 100% certainty in many different situations?”
In a way we can say, that a certain prediction is going to almost for certain become reality, but it is impossible to state the probability of that for sure. Or more specifically, the exact assumptions are always based on assuming, that the observations are going to match with a certain distribution.
In a way it’s not really relevant in any way, if we are getting 90% or 99% certainty about something, because we will never get the 100%.
It’s more relevant to think, what are the possible benefits of using the prediction, and what kind of damage it can do for us, if the prediction is not correct.
If the predictions are solely used to motivate sales, ergo for showing the vendor that if they just take in enough meetings they will make more sales, the possible downside to that prediction is practically non-existing.
However, if your whole customer acquisition is based on the assumption that these customers will bring in a lot of money in the long run, but the first six months end up being unprofitable, the falling through of that specific prediction will cause either bankruptcy, or at the very least more financing problems.
Roughly put, it is not smart to count on predictions on the long run, no matter how likely they might seem.
Sure there are grey areas to this, as well there is to everything, but if your business doesn’t have a monopoly, the market itself is so complicated, that one factor left out of the equation of making that prediction might wipe your whole business of the face of the Earth in a matter of months.
Usually, the entrepreneurs that have their own money invested in their business, act by this rule automatically. Employees with different roles to play however, can act on a completely separate logic, especially if their wages are tied to factors outside of the actual sales.
The more unstable the market and the longer the time span – the harder the prediction
As a rule of thumb when it comes to predictions is, that the more unstable the market and the longer the time span, the more difficult it is to make an accurate prediction.
The time span is usually very obvious one. It is fairly easy to predict sales within the next two weeks from now, by just looking at offers that have been sent to customers and the daily performance of the salesmen according to phone calls and agreed appointments.
To predict the sales a year from now… Now there lies the challenge.
But this doesn’t mean, that it’s impossible to predict the amount of sales with some certainty and even quite far ahead in the future.
If the amount of made phone calls correlates clearly with the sales, it is quite simple to come to the conclusion that to reach your growth goals. You need to recruit a certain amount of new salesmen.
The problem arises because there are of course differences between the salesmen. One salesman can make ten times the sales as another one does. And accordingly, the market can also change.
Suddenly the appointments are not turning into sales as they did before, and that’s why the predictions don’t hold either.
And this brings us to the topic of market instability. If the market is very stable and the demand doesn’t change much, the variables affecting the sales usually occur inside of the company, and that makes it easier to predict the company’s future.
And on the contrary, if the market is dependent on, for example the weather conditions, predicting the amount of sales becomes even more challenging.
As a good example of this type of case think about air conditioners – when the heat waves arrive in the Summer time, there will be for sure a big rise in the sales of air conditioners. But from the point of the vendor, it is impossible to predict what kind of weather conditions the Summer is going to bring.
Of course now somebody can claim, that it is actually possible to predict as far as six months ahead something as complicated as our weather conditions, but the truth is that the success rate of that prediction is hardly existing.
Should we even bother to try to predict the effectiveness of sales and marketing at all?
Yes, we should, BUT. You shouldn’t trust the predictions too much. It is very useful to know, that your best customers came from a Facebook campaign, and that their lifetime value costs X-amount of euros.
It is also very useful to remember, that this is not a guarantee for the future, and there are usually so many variables, that the predicting is practically impossible.
The main problem with the predictions is to figure out how much your company’s actions are affecting the accuracy of the prediction. If the factors outside the sphere of influence of your company, such as development of Google searches volumes only cause changes of few percents, the prediction can be on a quite firm ground.
Then of course, there can appear another factor outside of the control of the company, that will end up destroying the whole business.
And in case the factors outside of your company are causing tens of percents of volatility the predicting becomes a lot more difficult.
From here we are reaching a point that for the sake of the predictability the activities of the company should be shifted more towards a direction, where most of the variables are under the control of the company.
In my own opinion, this is one of the biggest factors that makes active sales, or, in other words, cold-calling, more worthwhile compared to inbound marketing.
Even with this blog we are of course using both inbound marketing and active sales, so these two don’t exclude one another.
However, the predictability of inbound marketing is much more complicated, especially in the beginning. Because, for an example, the company has very little control over the search volume of Google, whereas the amount of outgoing phone calls is a lot greater. On the contrary, the effectiveness of inbound marketing is a lot more difficult to predict in the long run.
On the other hand, separating inbound and outbound can be a bit forced and it can be unclear where to draw the line. For an example, Facebook’s paid advertisement is usually considered as inbound sales, even though in a way it’s more “pushing” and closer to traditional sales than search advertising is.
Through this, Facebook advertisement is in a way more predictable, because it is not dependent on search volumes, but instead it’s tied to people’s use of Facebook in general. You could claim, that use of Facebook is on a more stable ground, than a single product or searching for services on Google are.
The impact of scale on predictability
One option how to make the prediction in a certain way more accurate, is to zoom out from the situation.
What seems to be a significant change in the monthly demand, can be completely negligible on an annual basis, or for example when looking at a five year cycle.
Usually, when a short term prediction is not giving the correct results, it is worth the while to have a look at the situation on a longer time frame. Based on this, you will get a more clear picture of if you should be reacting to the situation in some way.
As an example, think about looking at the same road from 10 meters distance, or from 10km up in the air. What looks like a curve from the surface of the Earth, can look like a straight line at 10 kilometers altitude. And if you zoom out even further, even the smallest remaining curves will disappear.
One of the great problems of predictability is also related to this. Even if you do everything right in terms of sales, the stars may be aligned in such a bad position, that the end result will be catastrophically bad sales.
And on the contrary, even if everything is done badly from the beginning, you can still be making record months. The smaller the business, the more difficult it is to draw these assumptions based on the cause and effect -relation.
From here we get to a central point that confuses me quite often, that many times volatility is considered as a bigger risk, compared to the recurring revenue.
That means, that being an entrepreneur is considered more difficult to predict than being an employee is. Recurring revenue more predictable and project business less predictable. However, this is not exactly the case.
Short term stability vs. long term stability
When predicting and counting the risks, people usually confuse short term and long term volatilities. You can get a better picture of the problematics of the subject by comparing the risks of the employer, and an employee.
The income of an entrepreneur (especially a small entrepreneur) often fluctuates more than an employee’s income does. The employee receives a steady income from one month to another, and higher volatility is less common in their income (excluding vendors, for example).
However, an entrepreneur typically has more customers than an employee with only one “customer” to whom he is working for. If an entrepreneur loses one customer, the income rarely collapses to zero.
If, on the other hand, that only one “customer”, that is, the employer, decides to say that they do not need the services of the employee anymore, the income drops to practically down to zero.
Typically, this mismatch is further emphasized by the fact, that the constant low volatility forces the entrepreneur to invest in customer acquisition and the ability to acquire new customers. Whereas on the contrary, the employee does not necessarily have to practice selling himself for many years.
Thus the employee’s earnings may seem more predictable, but they are more predictable only until the employer tells you “by-bye”. When zooming out on a slightly larger scale, you can see that the entrepreneur’s income is actually often much more stable, than the employee’s income.
Comparing this same logic to the predictability and stability of a company’s business, there are three core questions:
- How many operators are the revenue of your company divided with, aka how dependent the company is of one client?
- How dependent on sales success is your invoicing?
- How much sales force does the organization hold, meaning, if X- % of the customers suddenly leave, how fast can this hole be patched?
This could still be accompanied by a fourth core question related to the sustainability of the companies’ volatility and, in particular, whether the business has a breaking point where the company goes bankrupt, if a sufficient number of customers go away, or the sale fails.
But as a topic this is in its own way completely different, so let’s just focus on these first three questions for now.
Usually when talking about business predictability, it is common knowledge that too much dependence on one customer should be avoided.
In addition, recurring revenue is generally perceived as a lower risk than the project business, and this is always the case when we are talking of a certain period of time.
In practice, a business model with recurring revenue makes the business more predictable, regardless of the success or failure of sales, whereas in the case of project sales the whole business is in a way ”at the mercy” of sales.
Typically the third question is left out of the equation, which is how much sales force the organization actually holds, if something surprising happens.
When running a project based business this doesn’t usually become a problem, because of the nature of the business it has a lot of sales force, and the appreciation of sales is very high.
However, there is usually less sales force in businesses with recurring revenue business models, and this is not even seen as a relevant concern. Because in the end, it is unlikely that 50 of our 150 customers would quit unexpectedly.
It is true that it is unlikely. Imagine, however, if there was a new innovation in your industry that would allow your customers to shift into using this new alternative as a mass, your business would not have much impact on the situation if you only have a single vendor, who is used to calling only hot leads. Apart from how fast you can create a product that is competitive with that new invention.
But, if there are 15 salesmen in your company, that are used to cold-calling, the reaction will be a lot more effective.
This is not exactly an argument against recurring revenue, but to point out an issue that seems to be forgotten more often in recurring revenue businesses than with project sales.
Project sales forces you to take this problem under consideration, whereas recurring revenue does not.
Therefore the situation with sales is partly the same as the case between the employee and the entrepreneur. The entrepreneur is used to selling, the employee often is not. Of course, the situation between companies is often not as apparent, as it is between entrepreneurs and employees.
Summary of the predictability of sales and marketing
We people are pretty bad at predicting anything. The shorter the time span and the greater the impact of our own actions have on the accuracy of the prediction, the better we are at predicting.
On the other hand, it is not so much about how good we are at predicting, but what kind of benefits we will receive when we are right about the predictions, and what type of harm they can cause to us, when we are wrong.
So it doesn’t matter if your prediction does not come true in the first hundred tries and you lose 10 € each time, if on the 101th prediction you get it right and you make 100,000 euros.
Finally, the main points to improve your business and business predictability are the following:
- Gain new customers and constantly develop your new customer acquisition.
- Strive to turn projects into continuous customerships (recurring revenue).
- Don’t get caught up with the short term transitions, but instead zoom out enough for being able to make the conclusion.
Compared to our general blog topics, this was perhaps a bit of an extraordinary subject and viewed from a slightly different angle, but hopefully this raised at least some thoughts about your own business.
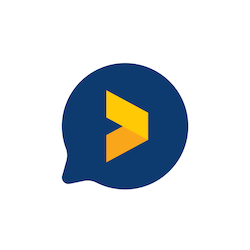