What Is Customer Sentiment? + 7 Ways To Analyze It
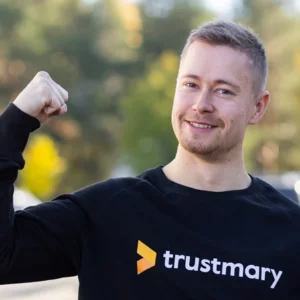
Last edited: March 14th, 2025
Understand your customers better, and turn happy customers into social proof for your website and social media.
Try Trustmary
Understanding customer sentiment has never been so important, helping to build a strong brand image that leads to a loyal customer base. With 66% of consumers saying that a bad experience can ruin their day, this shows just how much impact a negative brand interaction can have.
As businesses work around the clock to provide products and services that leave their customers feeling great, customer sentiment data is invaluable. With insights that reveal whether customers are showing positive or negative sentiment across different channels, it’s easier than ever to uncover pain points and provide a lasting solution.
In this article, I will explain what customer sentiment is and how to prepare data for a customer sentiment analysis.
I’ll then go over seven different ways that sentiment data can be analyzed to help businesses find the approach that helps them grow with customer insights.
What is Customer Sentiment?
Customer sentiment is a metric that refers to the emotions a consumer feels about a brand experience. It can be used to gain a deeper understanding of people’s feelings towards a product or service.
By being able to analyse large amounts of text data, including sources such as third-party reviews, customer service interactions, survey responses, and social media conversations, a business can gain valuable insights into customer sentiment. This can then be used to establish areas of strength and opportunities for improvement.
Customer sentiment is a crucial consideration for all modern businesses.
I’ve seen a few cases, where analyzing customer sentiment has led making significant changes to the whole digital customer experience, which leads to happier customers and less customer churn.
Can you afford not to understand your customers better?
Preparing Data For Customer Sentiment Analysis
To analyse customer sentiment, the data needs to be prepared. Here are some of the steps which can be taken to get the data ready:
1. Define the scoring system
To maintain consistency when tracking customer sentiment, a numerical scoring system needs to be put in place.
Some businesses choose to stick to a simple scale that adds +1 for positive sentiments, -1 for negative sentiments, or 0 for neutral sentiments. If a more detailed analysis is preferred to include more specific emotions, a wider scale can be implemented with different scores for each category.
2. Identify sentiments
Whether manually reviewing text or using machine learning to analyze large quantities of text, rules need to be implemented to determine what is classed as a positive or negative sentiment.
Predetermining words or phrases that are commonly associated with each emotion will help to group each piece of text accurately.
For example, negative keywords may include “poor quality” or “disappointing”, whereas positive keywords would look more like “easy to use” and “great”.
3. Gather all data sources
To avoid skewing results, pulling together data from all platforms will ensure a comprehensive picture of customer sentiment is given.
Bring together
- Third-party reviews from platforms such as Google and Facebook
- Social media conversations from active channels
- Customer service queries, tickets and discussions
- Any user-generated content, such as first-party reviews
- Customer feedback data
Monitoring all platforms will ensure that any trends across platforms can be established and any areas of concern can be worked on. Using web scraping tools, such as Web Scraper, will make the process easier and prevent duplicate data.
4. Implement a reporting strategy
To avoid losing track of where all of the raw files came from, using a customer reporting strategy will ensure the data is well-kept and clearly structured.
Having a written record of the feedback left by customers, along with the response given by the customer service team, will make it easier to track the interactions and identify areas for improvement.
Does that Sound Too Complicated?
Only huge companies with huge budgets can start such a big project from scratch.
Smaller companies should try importing their customer feedback data and third-party reviews into Trustmary.
We’ll help you keep track of customer sentiment and how it develops over time.
7 Ways To Analyse Customer Sentiment Data
After gathering customer sentiment data, choosing the best technique for analysing the data can make all the difference. Here are seven different methods which can be utilised:
1. Sentiment analysis
A sentiment analysis consists of categorising text into sentiments to monitor customer satisfaction.
By splitting all customer interactions into positive, negative, or neutral sentiments, it is easier than ever to track customer satisfaction and identify any peaks or dips.
There are various tools available to help carry out sentiment analysis, including Google Natural Language AI, which uses machine learning to analyse text and understand customer attitudes. This process is particularly effective when looking into large quantities of customer feedback over time.
2. Emotion detection
Emotion detection is a more detailed analysis technique that uses natural language processing to detect any emotional cues from text.
By being able to segment emotions, whether that be happiness, surprise, anger, or disappointment, a business is able to connect with its customers on a deeper level and adjust its marketing communications accordingly. This can then be used to get a deeper understanding of customer motivations and pain points.
To implement emotion detection, predefined words and phrases will need to be set to help identify the different emotions. Natural language processing models can be used to do this on a larger scale.
3. Aspect-based analysis
When it comes to product development, an aspect-based analysis can be carried out to break down customer opinions based on the nature of the feedback.
Once the different aspects have been defined, the analysis can be carried out to collate any feedback that fits into each category. Some examples of categories that might be used by a business include customer service, delivery, price, and quality.
This method is particularly useful when looking into the areas of the business that need improvement. It can also be used to break down feedback across different product categories – a powerful strategy when looking to create new products or adjust existing ones.
4. Intent analysis
An intent analysis is used to establish the reason for customer feedback, providing insight into the main topics that customers are discussing. Examples of intent would typically include complaints, inquiries, purchase intent, or compliments.
By having a clearer understanding of intent, the customer service team can be more prepared to address the feedback and identify any concerns.
This form of analysis is particularly insightful for websites that contain, or are looking to contain, a live chat function. By having more understanding of common queries and topics, the predefined answers can be tailored.

5. AI classification
Artificial intelligence (AI) can provide significant value when analysing customer sentiment data as vast amounts of data can be analysed in seconds.
Popular AI tools such as ChatGPT and Google Gemini are becoming more advanced at detecting human emotions. By analysing text, they are able to identify whether the customer feedback was written with emotions such as gratitude, disappointment, confusion, anger, or even sarcasm.
This strategy can be particularly effective when it comes to analysing large chunks of data or particularly long-form content, saving significant amounts of time in comparison to human analysis.
6. Topic modelling
Similarly to intent analysis, topic modelling can be used to identify key themes among customer feedback. However, instead of focusing on the discussion category, topic modelling looks for broader patterns without necessarily needing to know the exact intent.
This method provides the best results when looking to make strategic decisions, grouping together customer feedback that contains related words or phrases. This allows the business to see opinions on specific products without having to cypher through irrelevant text.
7. Multilingual analysis
For businesses that work on a global scale, different languages can make it difficult to carry out a regular analysis.
A multilingual customer sentiment analysis uses natural language processing models to group sentiments in different languages. By being able to interpret the sentiments after translation, feedback can be analysed on a broader scale to take the wider market into consideration.
It is also possible to break down the analysis by region, helping to understand any differences in pain points among different customer bases.
Using Feedback To Drive Growth
A customer sentiment analysis is a powerful strategy which helps achieve a better understanding of customer emotions and motivations. By grouping data based on positive, negative, and neutral sentiments, trends can be identified and action can be taken to improve the customer experience.
Collating data across third-party review sites, social media platforms, feedback forms, and customer service interactions will mean all brand-related feedback can be continually monitored and addressed to manage brand image and resolve any negative feedback.
By choosing the most appropriate analysis strategy to carry out a customer sentiment analysis, a business is able to tailor the approach to their personal needs and find a solution that will help them build stronger relationships among their customer base.
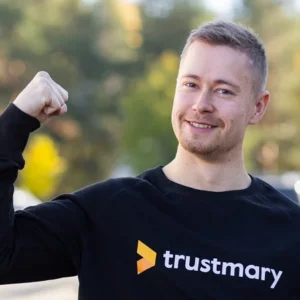
Pasi Isomäki
Pasi Isomäki is a Customer Success Manager at Trustmary. He is especially known for his ability to nurture customer relationships. With a background in Sales, he also knows how to win over new customers.